Improving multi-objective random one-bit climbers on MNK-landscapes
Abstract:
Multi-objective random one-bit climbers (moRBCs) are one class of stochastic local search-based algorithms that maintain a reference population of solutions to guide their search. They have been shown to perform well in solving multi-objective optimization problems. In this work, we further enhance the moRBCs by introducing tabu moves to improve their efficiency and search for more promising solutions. We also improve the selection to update the reference population and archive using a procedure that provides better mechanism to preserve diversity among the solutions. We use several MNK-landscape models to study the behavior of the modified moRBCs. © 2010 IEEE.
Año de publicación:
2010
Keywords:
- many-objective optimization
- MNK-Landscapes
- multi-objective optimization
- Random bit climbers
- Adaptive ε-ranking
Fuente:
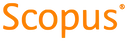
Tipo de documento:
Conference Object
Estado:
Acceso restringido
Áreas de conocimiento:
- Algoritmo
- Algoritmo
Áreas temáticas:
- Ciencias de la computación