Improving pbkp_rediction of students’ performance in intelligent tutoring systems using attribute selection and ensembles of different multimodal data sources
Abstract:
The aim of this study was to pbkp_redict university students’ learning performance using different sources of performance and multimodal data from an Intelligent Tutoring System. We collected and preprocessed data from 40 students from different multimodal sources: learning strategies from system logs, emotions from videos of facial expressions, allocation and fixations of attention from eye tracking, and performance on posttests of domain knowledge. Our objective was to test whether the pbkp_rediction could be improved by using attribute selection and classification ensembles. We carried out three experiments by applying six classification algorithms to numerical and discretized preprocessed multimodal data. The results show that the best pbkp_redictions were produced using ensembles and selecting the best attributes approach with numerical data.
Año de publicación:
2021
Keywords:
- Pbkp_redicting academic performance
- Intelligent Tutoring Systems
- multimodal learning
- data fusion
- Multisource data
Fuente:
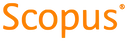
Tipo de documento:
Article
Estado:
Acceso abierto
Áreas de conocimiento:
- Aprendizaje automático
- Tecnología educativa
Áreas temáticas:
- Funcionamiento de bibliotecas y archivos