Improving the EDCM mixture model with expectation propagation
Abstract:
Bayesian inference is crucial to challenging scenarios that involve complex probabilistic models, which are usually intractable. In this work, we develop an expectation propagation approach to learn finite mixture models of EDCMs. The EDCM (Elkan 2006) is an exponential-family approximation to the widely used Dirichlet Compound Multinomial distribution and has been shown to offer excellent modeling capabilities in the case of sparse count data. Expectation propagation is a deterministic approach that provides accurate approximations to the full posterior and allows to include prior beliefs in the model as opposed to the maximum-likelihood method, which provides point estimates only. We evaluate the efficiency of our framework on several datasets for sentiment analysis and shape recognition. Our proposed model shows comparable to superior results to other approaches in the literature.
Año de publicación:
2020
Keywords:
Fuente:
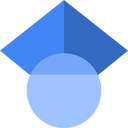
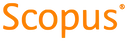
Tipo de documento:
Conference Object
Estado:
Acceso restringido
Áreas de conocimiento:
- Aprendizaje automático
- Algoritmo
Áreas temáticas:
- Programación informática, programas, datos, seguridad
- Métodos informáticos especiales
- Matemáticas