In Silico Pbkp_rediction of the Toxicity of Nitroaromatic Compounds: Application of Ensemble Learning QSAR Approach
Abstract:
In this work, a dataset of more than 200 nitroaromatic compounds is used to develop Quantitative Structure–Activity Relationship (QSAR) models for the estimation of in vivo toxicity based on 50% lethal dose to rats (LD50). An initial set of 4885 molecular descriptors was generated and applied to build Support Vector Regression (SVR) models. The best two SVR models, SVR_A and SVR_B, were selected to build an Ensemble Model by means of Multiple Linear Regression (MLR). The obtained Ensemble Model showed improved performance over the base SVR models in the training set (R2 = 0.88), validation set (R2 = 0.95), and true external test set (R2 = 0.92). The models were also internally validated by 5-fold cross-validation and Y-scrambling experiments, showing that the models have high levels of goodness-of-fit, robustness and pbkp_redictivity. The contribution of descriptors to the toxicity in the models was assessed using the Accumulated Local Effect (ALE) technique. The proposed approach provides an important tool to assess toxicity of nitroaromatic compounds, based on the ensemble QSAR model and the structural relationship to toxicity by analyzed contribution of the involved descriptors.
Año de publicación:
2022
Keywords:
- Accumulated Local Effect
- ensemble model
- nitroaromatic compounds
- Support Vector Machine
- QSTR
- QSAR
- Toxicity
- Machine learning
Fuente:
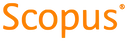
Tipo de documento:
Article
Estado:
Acceso abierto
Áreas de conocimiento:
- Relación cuantitativa estructura-actividad
- Aprendizaje automático
- Toxicología
Áreas temáticas:
- Química analítica