Increasing the Segmentation Accuracy of Aerial Images with Dilated Spatial Pyramid Pooling
Abstract:
This thesis addresses the environmental uncertainty in satellite images as a computer vision task using semantic image segmentation. We focus in the reduction of the error caused by the use of a singleenvironment models in wireless communications. We propose to use computer vision and image analysis to segment a geographical terrain in order to employ a specific propagation model in each segment of the link. Our computer vision architecture achieved a segmentation accuracy of 89.41%, 86.47%, and 87.37% in the urban, suburban, and rural classes, respectively. Results indicate that estimating propagation loss with our multi-environment model reduced the root mean square deviation (RMSD) with respect to two publicly available tracing datasets.
Año de publicación:
2020
Keywords:
- image analysis
- Supervised learning
- Computer Vision
- Wireless communications
- Pattern Recognition Image Segmentation
Fuente:
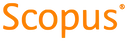
Tipo de documento:
Article
Estado:
Acceso abierto
Áreas de conocimiento:
- Visión por computadora
- Ciencias de la computación
- Simulación por computadora
Áreas temáticas:
- Métodos informáticos especiales