Inference for stochastic differential equations models using filtering algorithms
Abstract:
The practical use of stochastic models in areas of applied research such as: finance, infectious diseases dispersal, telecommunications, audio signal processing, optimum process control, machines of learning, global position systems, and physical systems, among other applications. Five filtering algorithms were implemented: extended Kalman filter (EKF), generic particle filter (GPF), particle filter with resampling (RPF), ensamble Kalman Filter (EnKF), and sigma-point Kalman filter (SPKF). The proposed algorithms allow the approximate reconstruction of the marginal distributions a posteriori of the unknown states of the system. The methodology is illustrated using two stochastic models that come from the world of finance. In the empirical study, it is shown that the filters have good performance in the estimation of the states. No significant differences were observed between the estimated values and the true values. The quality of estimation of the algorithms is evaluated by the goodnes of fit measure through the calculation of the root of the mean square error obtaining small estimation errors, in addition we estimated the execution times of the algorithms obtaining satisfactory results.
Año de publicación:
2017
Keywords:
Fuente:
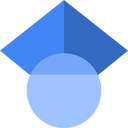
Tipo de documento:
Other
Estado:
Acceso abierto
Áreas de conocimiento:
- Proceso estocástico
- Optimización matemática
- Optimización matemática
Áreas temáticas:
- Análisis
- Probabilidades y matemática aplicada
- Ciencias de la computación