Information fusion to automatically classify post-event building damage state
Abstract:
Post-event reconnaissance missions are conducted after each major natural hazard event to collect valuable and perishable data. Teams of engineers and scientists are deployed to collect data, and in particular visual data (images), to support particular lines of inquiry, or to identify new lines of inquiry, that may lead to new knowledge about the best practices for the design of civil infrastructure. Visual data, combined with computer vision methods, can be a valuable tool for accelerating and automating these processes. Together they provide the means to more easily use the data, and organize the data sets so that they can be discovered in a search and reused. The focus of this paper is the development of an automated technique to classify the overall damage state of a building based on a typical set of reconnaissance images collected from a single building in the field. The motivating task is the collection of data and classification of damage into broad categories, such as those needed for computing the Hassan index (Pujol et al., 2020). The method adopts a naïve Bayes fusion algorithm (Altınçay, 2005) to combine the data, and an integrated sampling technique to reduce the computational time without compromising the quality of the results. Validation is performed using 29,543 past reconnaissance images from 720 buildings in different parts of the world that was collected, in part, for determination of the Hassan index.
Año de publicación:
2022
Keywords:
- Naïve Bayes fusion
- Information Fusion
- Post-event reconnaissance
- Building damage state classification
Fuente:
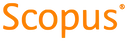
Tipo de documento:
Article
Estado:
Acceso restringido
Áreas de conocimiento:
- Análisis de datos
Áreas temáticas:
- Tecnología (Ciencias aplicadas)
- Ingeniería y operaciones afines
- Sistemas