Integrating artificial neural networks and cellular automata model for spatial-temporal load forecasting
Abstract:
The long-term distribution planning should include an understanding of consumer behavior and needs to develop strategic expansion alternatives that meet the future demand. The magnitude of growth along with the place where and when it will be developed are determined by the spatial load forecasting. Thus, this paper proposes a spatial-temporal load forecasting method to recognize and pbkp_redict development patterns using historical dynamics and determine the development of consumers and electric load in small areas. An artificial neural network is integrated to a cellular automaton method to establish transition rules, based on land-use preferences, neighborhood states, spatial constraints, and a stochastic disturbance. The main feature is the incorporation of temporality, as well as taking advantage of geospatial-temporal data analytics to calibrate and validate a holistic and integral framework. Validation consists of measuring the spatial error pattern during the training and testing phase. The performance of the method is assessed in the service area of an Ecuadorian power utility. The knowledge extraction from large-scale data, evaluating the sensitivity of parameters and spatial resolution was carried out in reasonable times. It is concluded that adequate normalization and use of temporality in the spatial factors improve the error in the spatial-temporal load forecasting.
Año de publicación:
2023
Keywords:
- Distribution planning
- Geospatial analysis
- Artificial Neural Network
- cellular automata
- Spatial Load Forecasting
- big data analytic
Fuente:
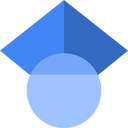
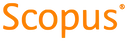
Tipo de documento:
Article
Estado:
Acceso restringido
Áreas de conocimiento:
- Aprendizaje automático
- Simulación por computadora
- Simulación por computadora
Áreas temáticas:
- Ciencias de la computación