Intelligent Health Indicators Based on Semi-supervised Learning Utilizing Acoustic Emission Data
Abstract:
Health indicators are indices that act as intermediary links between raw SHM data and prognostic models. An efficient HI should satisfy prognostic requirements such as monotonicity, trendability, and prognosability in such a way that it can be effectively used as an input in a prognostic model for remaining useful life estimation. However, discovering or designing a suitable HI for composite structures is a challenging task due to the inherent complexity of the evolution of damage events in such materials. Previous research has shown that data-driven models are efficient for accomplishing this goal. Large labeled datasets, however, are normally required, and the SHM data can only be labeled, respecting prognostic requirements, after a series of nominally identical structures are tested to failure. In this paper, a semi-supervised learning approach based on implicitly imposing prognostic criteria is adopted to design a novel HI suitable. To this end, single-stiffener composite panels were subjected to compression-compression fatigue loading and monitored using acoustic emission (AE). The AE data after signal processing and feature extraction were fused using a multi-layer LSTM neural network with criteria-based hypothetical targets to generate an intelligent HI. The results confirm the performance of the proposed scenario according to the prognostic criteria.
Año de publicación:
2023
Keywords:
- Semi-supervised deep neural network
- Intelligent health indicator
- Prognostic and health management
- Structural Health Monitoring
- Composite structures
Fuente:
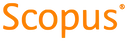
Tipo de documento:
Conference Object
Estado:
Acceso restringido
Áreas de conocimiento:
- Aprendizaje automático
- Ciencias de la computación
Áreas temáticas:
- Fisiología humana
- Métodos informáticos especiales
- Medicina y salud