Intelligent pbkp_redictive model of BMI in nutritionists' patients using Machine Learning algorithms: Logistic Regression and Neural Networks
Abstract:
The residents of the Bastión Popular Cooperative in Ecuador have health problems due to inadequate food due to limited economic resources, poor nutrition, and lack of knowledge about healthy food in the people of this vulnerable area. On the other hand, this area has a limited number of nutritionists to serve the population, for this reason it is necessary that the professional can serve them more quickly to be able to cover the largest number of them during the working day, in this situation this project proposes the creation of a technological solution to help the specialist to minimize the time spent on manual calculations made during the consultation. The nutritionist as part of the diet prescription process must consider several variables and conditions of the patient to establish the correct BMI (body mass index) of each type of patient, so this study promotes the creation of an intelligent model that allows the doctor to obtain the BMI in a more agile way, for this case qualitative, quantitative and experimental research was applied using Machine Learning algorithms, Multinomial Logistic Regression and Dense Layer Neural Networks. As results it was obtained that the model based on Multinomial Logistic Regression obtained an efficiency level of 97.9% in test data, while the model based on Neural Networks with dense layer obtained an efficiency level of 98.95% for test data. Therefore, it was found that the Neural Networks classifier allows the nutritionist to avoid manual calculations and instead obtain the BMI with a high level of efficiency, thus saving time in the initial phase of the patient consultation, giving him/her the opportunity to use that time to attend more patients in the waiting room.
Año de publicación:
2022
Keywords:
- Dense Neural Networks
- Supervised learning
- Multiclass
- Machine learning
- Multinomial Logistic Regression
Fuente:
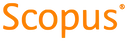
Tipo de documento:
Conference Object
Estado:
Acceso abierto
Áreas de conocimiento:
- Aprendizaje automático
- Nutrición
- Algoritmo
Áreas temáticas:
- Ciencias de la computación