Intelligent sensor validation for sustainable influent quality monitoring in wastewater treatment plants using stacked denoising autoencoders
Abstract:
Wastewater treatment plants (WWTPs) influent conditions can dramatically affect a treatment unit's state and effluent quality. WWTP sensors may record faulty measurements due to abnormal events or the malfunction of the system, leading to serious problems in the system's operation and the violation of effluent discharge standards. Therefore, automatic fault detection and faulty data reconciliation are crucial for an efficient and stable WWTP monitoring. In this study, a holistic framework for sensor validation of WWTP influent conditions is presented considering the non-linearity, measurement noise, and complexity of the WWTP's data. A stacked denoising autoencoder (SDAE) model is proposed to detect, identify, and reconcile faulty data based on data from a real WWTP in South Korea. The proposed SDAE architecture presented a detection rate (DR) between 74% and 98%. The faulty sensor was identified using an SDAE-based sensor validity index (SVI). Data reconciliation showed that the SDAE was the most suitable reconciliation method based on the root mean square error (RMSE) for total nitrogen (TN) influent conditions of 4.04 mg N/L. Finally, faulty, noisy, and reconciled measurements were evaluated in a WWTP model to determine the proposed method's resilience potential.
Año de publicación:
2021
Keywords:
- Stacked denoising autoencoder
- Smart monitoring
- Wastewater treatment
- Fault Detection
- Sensor validation
Fuente:
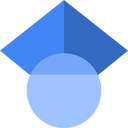
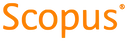
Tipo de documento:
Article
Estado:
Acceso restringido
Áreas de conocimiento:
- Ciencias de la computación
- Ingeniería ambiental
- Aprendizaje automático
Áreas temáticas de Dewey:
- Física aplicada
- Ingeniería sanitaria

Objetivos de Desarrollo Sostenible:
- ODS 6: Agua limpia y saneamiento
- ODS 12: Producción y consumo responsables
- ODS 9: Industria, innovación e infraestructura
