Interactive parameter tuning of bi-objective optimisation algorithms using the empirical attainment function
Abstract:
We propose a visual approach of eliciting preferences from a Decision Maker (DM) in the context of comparing the stochastic outcomes of two alternative designs or parameter configurations of an optimization algorithm for bi-objective problems. Our proposal is based on visualizing the differences between the empirical attainment functions (EAFs) of the two alternative algorithmic configurations, and then ask the DM to choose their preferred side of the differences. Information about the regions preferred by the DM is translated into a weighted hypervolume indicator that will assign higher quality values to approximation fronts that result in EAF differences preferred by the DM. This indicator may be used to guide an automatic algorithm configuration method, such as irace, to search for parameter values that perform better in the objective space regions preferred by the DM. Experiments on the well-known bi-objective quadratic assignment problem and a real-world production planning problem arising in the manufacturing industry show the benefits of the proposal. This manuscript for the Hot-off-the-Press track at GECCO 2021 is based on the paper: "Incorporating Decision-Maker's Preferences into the Automatic Configuration of Bi-Objective Optimisation Algorithms"published by European Journal of Operational Research [6].
Año de publicación:
2021
Keywords:
- empirical attainment function
- Multi-objective optimisation
- Automatic algorithm design and configuration
- Decision maker's preferences
Fuente:
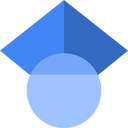
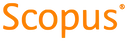
Tipo de documento:
Conference Object
Estado:
Acceso abierto
Áreas de conocimiento:
- Optimización matemática
- Optimización matemática
- Optimización matemática
Áreas temáticas:
- Ciencias de la computación