Inventory Control under Unobserved Losses with Latent State Learning
Abstract:
The unobserved losses have been a challenge to the applicability of automatic systems for inventory control. Due to the inability to record these events, systems cannot make the best decisions. In this sense, it is necessary to account for these losses to learn policies that allow inventory to be managed automatically. In this work, reinforcement learning has been successfully applied to an inventory management problem with unobserved losses. To do so, an inventory level inference model that considers unobserved losses was used. This solution does not depend on any distribution assumptions over sales and unobserved losses, but rather it estimates the distributions solely from observations and inputs of the system. During training, the model learns the transition and observation functions as distributions over inventory levels. The model simultaneously accounts state uncertainty and computes beliefs over inventory states. After the model is trained, it can be combined with a model-free reinforcement learning algorithm, such as Q-learning, to determine a policy for the inventory management. This policy can be used as automated system for the management of the inventory. The results suggests that this system can improve the inventory management performance by reducing the uncertainty of inventory levels without specifying the transition and observation distributions.
Año de publicación:
2021
Keywords:
- inventory inference
- state uncertainty
- recurrent state space model
Fuente:
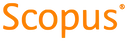
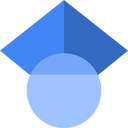
Tipo de documento:
Conference Object
Estado:
Acceso restringido
Áreas de conocimiento:
- Aprendizaje automático
- Optimización matemática
Áreas temáticas de Dewey:
- Dirección general
- Probabilidades y matemática aplicada
- Métodos informáticos especiales

Objetivos de Desarrollo Sostenible:
- ODS 12: Producción y consumo responsables
- ODS 8: Trabajo decente y crecimiento económico
- ODS 9: Industria, innovación e infraestructura
