Kernel Learning by Spectral Representation and Gaussian Mixtures
Abstract:
One of the main tasks in kernel methods is the selection of adequate mappings into higher dimension in order to improve class classification. However, this tends to be time consuming, and it may not finish with the best separation between classes. Therefore, there is a need for better methods that are able to extract distance and class separation from data. This work presents a novel approach for learning such mappings by using locally stationary kernels, spectral representations and Gaussian mixtures.
Año de publicación:
2023
Keywords:
- approximating kernel
- non-parametric kernel learning
- locally stationary kernel
- kernel spectral representation
Fuente:
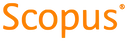
Tipo de documento:
Article
Estado:
Acceso abierto
Áreas de conocimiento:
- Aprendizaje automático
Áreas temáticas de Dewey:
- Ciencias de la computación
- Lengua
- Física aplicada