Kernel-Spectral-Clustering-Driven Motion Segmentation: Rotating-Objects First Trials
Abstract:
Time-varying data characterization and classification is a field of great interest in both scientific and technology communities. There exists a wide range of applications and challenging open issues such as: automatic motion segmentation, moving-object tracking, and movement forecasting, among others. In this paper, we study the use of the so-called kernel spectral clustering (KSC) approach to capture the dynamic behavior of frames - representing rotating objects - by means of kernel functions and feature relevance values. On the basis of previous research works, we formally derive a here-called tracking vector able to unveil sequential behavior patterns. As a remarkable outcome, we alternatively introduce an encoded version of the tracking vector by converting into decimal numbers the resulting clustering indicators. To evaluate our approach, we test the studied KSC-based tracking over a rotating object from the COIL 20 database. Preliminary results produce clear evidence about the relationship between the clustering indicators and the starting/ending time instance of a specific dynamic sequence.
Año de publicación:
2019
Keywords:
- Spectral clustering
- motion tracking
- Kernels
Fuente:
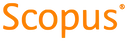
Tipo de documento:
Conference Object
Estado:
Acceso restringido
Áreas de conocimiento:
- Aprendizaje automático
- Simulación por computadora
- Simulación por computadora
Áreas temáticas:
- Métodos informáticos especiales