LAN Network Optimization after a DDoS Attack Detected with Supervised Learning
Abstract:
The Distributed Denial of Service (DDoS) attack is one of the most dangerous cyberattacks on the Internet, so can affect any server on any type of network, causing connectivity problems and even total loss of services. Machine learning can solve computational security problems and is frequently used to defend against cyber attacks. This article proposes the construction of a network topology where several DDoS attacks were applied, which will be detected by three Machine Learning classification algorithms. A dataset was generated from the collection of packets circulating in the network with samples of normal traffic and malicious packets, on which the experimental tests were carried out. In the classification task, the best performing supervised learning algorithm was Random Forest, with an accuracy of 100%. Finally, upon detecting a DDoS attack on the network, Dijkstra's optimization algorithm is applied to find an alternative route to mitigate network oversaturation. Two scenarios were proposed, the first analyzes the optimal route in an attacked network and the second without attacks. The results show a reconfiguration in the network to avoid routes where DDoS attack detection was applied.
Año de publicación:
2022
Keywords:
- random forest
- Support Vector Machine
- CYBERSECURITY
- malicious traffic detection
- logistic regression
- Dijkstra Algorithm
- Machine learning
Fuente:
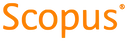
Tipo de documento:
Conference Object
Estado:
Acceso restringido
Áreas de conocimiento:
- Aprendizaje automático
- Ciencias de la computación
Áreas temáticas de Dewey:
- Ciencias de la computación

Objetivos de Desarrollo Sostenible:
- ODS 9: Industria, innovación e infraestructura
- ODS 17: Alianzas para lograr los objetivos
- ODS 8: Trabajo decente y crecimiento económico
