LTI ODE-valued neural networks: Multiple problem solving using a single neural structure
Abstract:
A dynamical version of the classical McCulloch & Pitts' neural model is introduced in this paper. In this new approach, artificial neurons are characterized by: i) inputs in the form of differentiable continuous-time signals, ii) linear time-invariant ordinary differential equations (LTI ODE) for connection weights, and iii) activation functions evaluated in the frequency domain. It will be shown that this new characterization of the constitutive nodes in an artificial neural network, namely LTI ODE-valued neural network (LTI ODEVNN), allows solving multiple problems at the same time using a single neural structure. Moreover, it is demonstrated that LTI ODEVNNs can be interpreted as complex-valued neural networks (CVNNs). Hence, research on this topic can be applied in a straightforward form. Standard boolean functions are implemented to illustrate the operation of LTI ODEVNNs. Concluding the paper, several future research lines are highlighted, including the need for developing learning algorithms for the newly introduced LTI ODEVNNs. © 2014 Springer Science+Business Media New York.
Año de publicación:
2014
Keywords:
- Parallel problem solving
- Dynamical neural network
- Complex-valued neural network
Fuente:
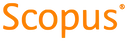
Tipo de documento:
Article
Estado:
Acceso restringido
Áreas de conocimiento:
- Red neuronal artificial
- Optimización matemática
- Optimización matemática
Áreas temáticas de Dewey:
- Ciencias de la computación