A 2-step algorithm for the estimation of time-varying single particle tracking models using Maximum Likelihood
Abstract:
Single particle tracking (SPT) is a powerful class of methods for studying the dynamics of biomolecules inside living cells. The techniques reveal both trajectories of individual particles, with a resolution well below the diffraction limit of light, and the parameters defining the motion model, such as diffusion coefficients and confinement lengths. Existing algorithms assume these parameters are constant throughout an experiment. However, it has been demonstrated that they often vary with time as the tracked particles move through different regions in the cell or as conditions inside the cell change in response to stimuli. In this work we apply the method of local Maximum Likelihood (ML) estimation to the SPT application combined with change detection. Local ML uses a sliding window over the data, estimating the model parameters in each window. Once we have found the values for the parameters before and after the change, we apply offline change detection to know the exact time of the change. Then, we reestimate these parameters and show that there is an improvement in the estimation of key parameters found in SPT. Preliminary results using simulated data with a basic diffusion model with additive Gaussian noise show that our proposed algorithm is able to track abrupt changes in the parameters as they evolve during a trajectory.
Año de publicación:
2019
Keywords:
Fuente:
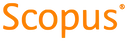
Tipo de documento:
Conference Object
Estado:
Acceso restringido
Áreas de conocimiento:
- Algoritmo
- Algoritmo
- Algoritmo
Áreas temáticas:
- Programación informática, programas, datos, seguridad
- Probabilidades y matemática aplicada
- Tecnología (Ciencias aplicadas)