A Benchmarking study of clustering techniques applied to a set of characteristics of MOOC participants
Abstract:
Massive Open Online Courses (MOOC) format is characterized by the great diversity of enrolled people. Moreover, the lack of prior knowledge of their profiles constitutes an important barrier with a view to identifying and getting a better understanding of underlying relationships in the internal structure of the features that make up the profile of the participants in those courses. This paper has the aim of identifying and analyzing the feasible set of MOOC participants' profiles by running two unsupervised clustering techniques, K-Means as a partitional clustering algorithm and Kohonen's Self-Organizing Maps (SOMs), hereinafter SOM, as a representative technique of Artificial Neural Networks (ANNs). The selected dataset for this paper comes from the MOOCKnowledge project data collection, which provides an opportunity to work with real-world data from hundreds of people. K-Means and SOM algorithms are performed with a subset of participants' features as input data. The clustering evaluation, meanwhile, is achieved with a selection of indices, an intra-cluster measure and an overall quality criterion for K-Means, and two measures related to topological ordering for SOM. The comparison of internal structure of both clustering (set of profiles) shows that there are similarities between them on the one hand and some pinpointed differences that can not be evaluated in advance without the opinion of an expert familiarized with the specifications of the MOOC on the other. Therefore, this comparison can not be considered conclusive until after a preliminary study of the results of the clustering interpretation for both algorithms. Finally, although it is not determined the clustering that best fits between K-Means and SOM, this study might help to provide a methodological guide on how to identify and select the appropriate clustering according to several quality criteria.
Año de publicación:
2016
Keywords:
- Clustering
- K-Means
- MOOC profiles
- CLÚSTER ANALYSIS
- Kohonen's self-organizing maps
- SOM
Fuente:
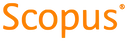