A Better Infected Hosts Detection Combining Ensemble Learning and Threat Intelligence
Abstract:
Ensemble learning techniques have been successfully proposed and used to improve threats detection in cybersecurity. These techniques usually improve the detection results by combining algorithms that together have less errors. However there has not been any ensemble learning algorithm used to classify network flows when several methods are used to give individual detections for each of the flows. The state of the art in the use of ensemble learning techniques was analyzed to find an alternative for the current intrusion detection mechanisms. This research proposes to incorporate ensemble learning to the Stratosphere Linux IPS (SLIPS), a behavioral-based intrusion detection and prevention system that uses machine learning algorithms to detect malicious behaviors. Our ensembling method is used to obtain better results, taking advantage of the benefits of SLIPS’s classifiers and modules. A contribution of our method is to extend the ensembling techniques by considering Threat Intelligence blacklists feeds as part of the detections. We present the results of the first stage of this project, i.e. ensemble learning algorithms to classify individual flows when they have multiple labels. on the other hand we also present the results corresponding to the second stage of our project, i.e. the detection of groups of flows going to the same destination IP.
Año de publicación:
2020
Keywords:
- intrusion detection
- CYBERSECURITY
- ensemble learning
- Malware
Fuente:
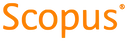
Tipo de documento:
Conference Object
Estado:
Acceso restringido
Áreas de conocimiento:
- Aprendizaje automático
- Ciencias de la computación
Áreas temáticas:
- Ciencias de la computación
- Ciencias políticas (Política y gobierno)
- Dirección general