A Comparative Study of Reinforcement Learning Algorithms Applied to Medical Image Registration
Abstract:
Nowadays, several medical procedures depend on the comparison and combination of images obtained in different modalities (magnetic resonance, computed tomography, PET, among others). Image registration is a geometric transformation process to align two or more images. It is necessary to have robust algorithms to find the best parameters of transformation in order to achieve accurate registrations. Reinforcement learning allows to train an agent through direct environment interaction, to achieve a goal. In this work, a comparison of the performance of Q-learning and Deep-Q with its variants is presented. Brain magnetic resonance images are used in 2D domain considering rigid deformations. The comparison is based on the reward values, computing the Pearson correlation factor in monomodal registration and Mutual information in multimodal registration, obtained during the learning process. It is also considered an error measure between the target parameters and the achieved ones. Finally, a backup memory criterion is proposed to train the Q-Network methods. Experimental results show a successfully behavior in all cases, but performance is improved when the proposed criterion is applied.
Año de publicación:
2020
Keywords:
- Q-Learning
- Machine learning
- Image registration
- reinforcement learning
- Deep-Q Networks
Fuente:
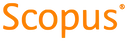
Tipo de documento:
Conference Object
Estado:
Acceso restringido
Áreas de conocimiento:
- Aprendizaje automático
- Laboratorio médico
- Ciencias de la computación
Áreas temáticas de Dewey:
- Ciencias de la computación
- Medicina y salud
- Enfermedades

Objetivos de Desarrollo Sostenible:
- ODS 3: Salud y bienestar
- ODS 4: Educación de calidad
- ODS 9: Industria, innovación e infraestructura
