A Comparison of Machine Learning Algorithms to Pbkp_redict Cervical Cancer on Imbalanced Data
Abstract:
Cervical cancer is a leading cause of death in women. The present research analyzes, explores, compares and identifies the best method for pbkp_redicting cervical cancer by applying machine learning techniques. The data is from the University Hospital of Caracas, Venezuela where a selection of variables was made according to the literature in order to pbkp_redict cervical cancer. Seven algorithms were applied: decision tree (DT), random forest (RF), logistic regression (LR), XGBoost (XG), Naive Bayes (NB), multilayer perceptron (MLP) and K-nearest neighbors (KNN). Furthermore, three imbalanced data techniques were applied: SMOTETomek, SMOTE, and ROS for Hinselmann, Schiller, Cytology and Biopsy as target variables. In addition, accuracy, precision, recall, f-score and AUC were used to evaluate the results. Random forest was the algorithm with the highest results in accuracy, precision and f-score, with 94.57%, 72.46% and 60.70% respectively. Logistic regression and Naive Bayes had the highest values for recall and AUC with 68.37% and 79.11% respectively.
Año de publicación:
2023
Keywords:
- Machine learning
- pbkp_rediction
- Imbalanced data techniques
- Cervical Cancer
Fuente:
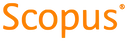
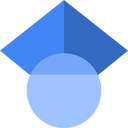
Tipo de documento:
Conference Object
Estado:
Acceso restringido
Áreas de conocimiento:
- Aprendizaje automático
Áreas temáticas:
- Ciencias de la computación
- Tecnología (Ciencias aplicadas)
- Medicina y salud