Large-scale nonlinear programming using IPOPT: An integrating framework for enterprise-wide dynamic optimization
Abstract:
Integration of real-time optimization and control with higher level decision-making (scheduling and planning) is an essential goal for profitable operation in a highly competitive environment. While integrated large-scale optimization models have been formulated for this task, their size and complexity remains a challenge to many available optimization solvers. On the other hand, recent development of powerful, large-scale solvers leads to a reconsideration of these formulations, in particular, through development of efficient large-scale barrier methods for nonlinear programming (NLP). As a result, it is now realistic to solve NLPs on the order of a million variables, for instance, with the IPOPT algorithm. Moreover, the recent NLP sensitivity extension to IPOPT quickly computes approximate solutions of perturbed NLPs. This allows on-line computations to be drastically reduced, even when large nonlinear optimization models are considered. These developments are demonstrated on dynamic real-time optimization strategies that can be used to merge and replace the tasks of (steady-state) real-time optimization and (linear) model predictive control. We consider a recent case study of a low density polyethylene (LDPE) process to illustrate these concepts. © 2008 Elsevier Ltd. All rights reserved.
Año de publicación:
2009
Keywords:
- Real-time optimization
- nonlinear programming
- Moving horizon estimation
- sensitivity
- Model Pbkp_redictive Control
Fuente:
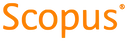
Tipo de documento:
Article
Estado:
Acceso restringido
Áreas de conocimiento:
- Optimización matemática
- Optimización matemática
- Optimización matemática
Áreas temáticas de Dewey:
- Programación informática, programas, datos, seguridad
- Ciencias de la computación
- Dirección general

Objetivos de Desarrollo Sostenible:
- ODS 9: Industria, innovación e infraestructura
- ODS 12: Producción y consumo responsables
- ODS 8: Trabajo decente y crecimiento económico
