A DNN approach to improving the short-term investment criteria for S&P500 index stock market
Abstract:
The investment decision criteria for the S&P500 Index stock market have been a challenge because the data is massive, complex, non-linear and noised. This study proposes the following approach: first, the Index data constitutes the input for training a Deep Learning Neural Network (DNN) for representing it adequately; and second, using the trained model and a sliding window technique, forecast short-term step by step stock values. The process takes into account a heuristic to control the possible extrapolation anomalies of the DNN. Finally, the generated information allows a supported decision. The representation of quantitative correlation values of S&P 500 Index data and their forecasting are promising. Qualitative options of the pbkp_rediction, constitutes decision making information. The present research permits academics to revise a new experience in data analysis; and, for practitioners, contributes to support investment decisions in the stock market.
Año de publicación:
2019
Keywords:
- S&P500 index
- stock market
- Deep learning neural network
- forecasting
Fuente:
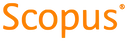
Tipo de documento:
Conference Object
Estado:
Acceso restringido
Áreas de conocimiento:
- Finanzas
- Aprendizaje automático
- Ciencias de la computación
Áreas temáticas:
- Economía financiera
- Métodos informáticos especiales