Learning Bayesian network by a mesh of points
Abstract:
In this work we applied Variable Mesh Optimization population metaheuristc (VMO) for Bayesian network (BN) structure learning as a score-and-search method. Our idea was to represent each node of the Mesh as a Bayesian network through a set of arcs. Then new BNs are created using (union and difference) operations among sets. For this process, three types of BNs are identified, local optima (BNs with the best score in each neighborhood), global optima (BN with the best score among local optima), and frontier solutions (most and least different in structure BNs). Finally the clearing process is applied to select the most representative BNs in the Mesh (score and structure). For determining the global score, each BN is used as a Bayesian classifier and classification accuracy is obtained using cross validation over dataset. The proposal is compared with other classifiers using UCI repository data set. Results show that our proposal obtains the best score, that proves to be a very competitive algorithm for supervised classification.
Año de publicación:
2016
Keywords:
Fuente:
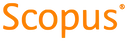
Tipo de documento:
Conference Object
Estado:
Acceso restringido
Áreas de conocimiento:
- Aprendizaje automático
- Optimización matemática
- Inferencia bayesiana
Áreas temáticas de Dewey:
- Ciencias de la computación

Objetivos de Desarrollo Sostenible:
- ODS 4: Educación de calidad
- ODS 17: Alianzas para lograr los objetivos
- ODS 9: Industria, innovación e infraestructura
