Learning Upper-Level Policy using Importance Sampling-based Policy Search Method
Abstract:
Policy search methods are a successful approach to reinforcement learning. These allow to learn upper-level policies whose main advantage is that these distributions explore directly in the parameter space. The contribution of this paper is to propose an algorithm based on importance sampling methods and local linear regression that uses the samples in an efficient way. In order to get this aim, we propose to include information of all the past samples in the learning process using importance sampling methods. Additionally, we use the gradient direction of the linear local model reward to explore regions where the pbkp_rediction of the reward could be better.
Año de publicación:
2018
Keywords:
Fuente:
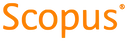
Tipo de documento:
Conference Object
Estado:
Acceso restringido
Áreas de conocimiento:
- Aprendizaje automático
- Política pública
Áreas temáticas:
- Programación informática, programas, datos, seguridad
- Ciencias sociales
- Matemáticas