Learning an Improved LMI Controller Based on Takagi-Sugeno Models via Value Iteration
Abstract:
This article proposes an alternative for formulating the method to improve the conservative controllers based on linear matrix inequality (LMI), action-value function (Q-function), and value iteration algorithm to learn optimal controllers by using system data. In this respect, the proposed uses ideas of the previous works that parametrize in a particular way the Q-function. In this sense, the Q-function can be described with polynomials membership functions for fuzzy models of Takagi-Sugeno and initialize a learning process with the LMI controller. The obtained controller uses both the information about the membership functions and a set of data obtained from the system to improve the LMI controller. A TORA system is used to illustrate the approach.
Año de publicación:
2022
Keywords:
- Takagi-Sugeno fuzzy models
- approximate dynamic programming
- Q function
- reinforcement learning
- linear matrix inequalities
Fuente:
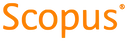
Tipo de documento:
Conference Object
Estado:
Acceso restringido
Áreas de conocimiento:
- Sistema de control
- Teoría de control
Áreas temáticas:
- Ciencias de la computación
- Lingüística aplicada
- Física aplicada