Learning non-linear time-scales with kernel γ-filters
Abstract:
A family of kernel methods, based on the γ-filter structure, is presented for non-linear system identification and time series pbkp_rediction. The kernel trick allows us to develop the natural non-linear extension of the (linear) support vector machine (SVM) γ-filter [G. Camps-Valls, M. Martínez-Ramón, J.L. Rojo-Álvarez, E. Soria-Olivas, Robust γ-filter using support vector machines, Neurocomput. J. 62(12) (2004) 493-499.], but this approach yields a rigid system model without non-linear cross relation between time-scales. Several functional analysis properties allow us to develop a full, principled family of kernel γ-filters. The improved performance in several application examples suggests that a more appropriate representation of signal states is achieved. © 2008 Elsevier B.V. All rights reserved.
Año de publicación:
2009
Keywords:
- Support Vector Machine
- Gamma filter
- Kernel
- Non-linear system identification
Fuente:
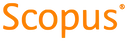
Tipo de documento:
Article
Estado:
Acceso restringido
Áreas de conocimiento:
- Aprendizaje automático
- Optimización matemática
Áreas temáticas:
- Ciencias de la computación