Learning sequences of sparse correlated patterns using small-world attractor neural networks: An application to traffic videos
Abstract:
The goal of this work is to learn and retrieve a sequence of highly correlated patterns using a Hopfield-type of attractor neural network (ANN) with a small-world connectivity distribution. For this model, we propose a weight learning heuristic which combines the pseudo-inverse approach with a row-shifting schema. The influence of the ratio of random connectivity on retrieval quality and learning time has been studied. Our approach has been successfully tested on a complex pattern, as it is the case of traffic video sequences, for different combinations of the involved parameters. Moreover, it has demonstrated to be robust with respect to highly variable frame activity. © 2011 Elsevier B.V.
Año de publicación:
2011
Keywords:
- Temporal sequence
- small-world
- Video retrieval
- Traffic analysis
- Attractor network
- Correlated patterns
- Sparse-coding
Fuente:
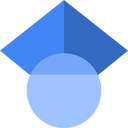
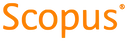
Tipo de documento:
Article
Estado:
Acceso restringido
Áreas de conocimiento:
- Aprendizaje automático
- Ciencias de la computación
- Ciencias de la computación
Áreas temáticas:
- Ciencias de la computación