Learning to Communicate with Autoencoders: Rethinking Wireless Systems with Deep Learning
Abstract:
The design and implementation of conventional communication systems are based on strong probabilistic models and assumptions. These fixed and conventional communication theories exhibit limitations in the utilization of the limited spectrum resources and the complexity of optimization for emerging wireless applications. Currently, new generations of wireless systems supported by artificial intelligence can learn from the wireless spectrum data, and optimize their utilization to enhance their performance. In this paper, we describe how deep learning can be used to design an end-to-end communication system using an encoder to replace the transmitter tasks such as modulation and coding, and a decoder for the receiver tasks such as demodulation and decoding. This flexible design can capture channel impairments effectively and optimize the operations of the transmitter and receiver altogether. We evaluate the case of a single-antenna system, incorporating impairments in the channel layer of the auto encoder and evaluating the response of different neural network optimization algorithms.
Año de publicación:
2020
Keywords:
- Autoencoders
- Wireless systems
- deep learning
- Channel estimation
- physical layer
Fuente:
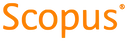
Tipo de documento:
Conference Object
Estado:
Acceso restringido
Áreas de conocimiento:
- Aprendizaje automático
- Comunicación
Áreas temáticas:
- Ciencias de la computación