A Deep Convolutional Autoencoder Architecture for Automatic Image Colorization
Abstract:
The inherent complexity of image colorization has motivated computer scientists towards the development of algorithms capable of simplifying the image colorization process. Despite the numerous advancements yielded by these efforts, there are still some limitations regarding the resulting image quality and its similarity to the ground truth counterpart. This paper proposes and implements a deep convolutional autoencoder architecture that maximizes the image colorization performance on two different datasets, the Fruit-360 and Flickr-Faces-HQ. To this end, a modification of the VGG16 model and a custom deep CNN model were assembled to predict and portray colors on grayscale images. We obtained mean absolute and square error results under the 0.01% on both datasets, demonstrating the substantial similarity between the output image and its ground truth counterpart. Additionally, the peak signal-to-noise ratio results of 27.72 (Fruits-360) and 26.86 (Flickr-Faces-HQ) indicate that the image colorization process introduces a relatively low drop in image quality.
Año de publicación:
2022
Keywords:
- deep convolutional autoencoder
- Image colorization
- VGG
- cnn
Fuente:
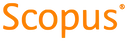
Tipo de documento:
Conference Object
Estado:
Acceso restringido
Áreas de conocimiento:
- Aprendizaje automático
- Ciencias de la computación
- Computadora
Áreas temáticas de Dewey:
- Métodos informáticos especiales