Long short-term memory networks for real-time runoff forecasting using remotely sensed data
Abstract:
Current efforts on Deep Learning-based modeling are being put for solving real world problems with complex or even not-fully understood interactions between pbkp_redictors and target variables. A special artificial neural network, the Long Short-Term Memory (LSTM) is a promising data-driven modeling approach for dynamic systems yet little has been explored in hydrological applications such as runoff forecasting. An aditional challenge to the forecasting task arises from the uncertainties generated when using readily-available Remote Sensing (RS) imagery aimed to overcome lack of in-situ data describing the runoff governing processes. Here, we proposed a runoff forecasting framework for a 300-km2 mountain catchment located in the tropical Andes of Ecuador. The framework consists on real-time data acquisition, preprocessing and runoff forecasting for lead times between 1 and 12 hours. LSTM models were …
Año de publicación:
2021
Keywords:
Fuente:
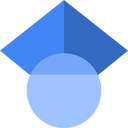
Tipo de documento:
Other
Estado:
Acceso abierto
Áreas de conocimiento:
- Aprendizaje automático
- Simulación por computadora
- Hidrología
Áreas temáticas:
- Ciencias de la computación