Low-cost deep learning-based architecture for detecting cardiac arrhythmias in ECG signals
Abstract:
This paper focuses on the development of a low-cost electrocardiographic device for the timely detection of cardiac arrhythmias such as tachycardia and bradycardia; disorders that can occur sporadically in a person's daily life. The architecture of the system for the classification of electrocardiogram (ECG) beats is based on a deep learning approach developed in Python 3 with low-cost and open-source elements. The training of the network is based on the background of the MIT-BIH database based on the AAMI and focused on 5 categories of cardiac beats of patients. The 1D convolutional neural network model was trained and validated using the TensorFlow and Keras libraries with an efficiency of 98%. The convolutional network tests are continuously monitored in real time in order to present the patient with the appropriate diagnosis or treatment. In case of any abnormality, an alert message is sent to the physician via a mobile application.
Año de publicación:
2022
Keywords:
- electrocardiographic
- Low-cost
- Architecture
- cardiac
- Arrhythmias
Fuente:
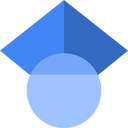
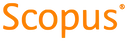
Tipo de documento:
Conference Object
Estado:
Acceso restringido
Áreas de conocimiento:
- Ciencias de la computación
- Enfermedad cardiovascular
- Aprendizaje automático
Áreas temáticas:
- Enfermedades
- Medicina y salud
- Ciencias de la computación