MACFE: A Meta-learning and Causality Based Feature Engineering Framework
Abstract:
Feature engineering has become one of the most important steps to improving model pbkp_rediction performance, and producing quality datasets. However, this process requires non-trivial domain knowledge which involves a time-consuming task. Thereby, automating such processes has become an active area of research and interest in industrial applications. In this paper, a novel method, called Meta-learning and Causality Based Feature Engineering (MACFE), is proposed; our method is based on the use of meta-learning, feature distribution encoding, and causality feature selection. In MACFE, meta-learning is used to find the best transformations, then the search is accelerated by pre-selecting “original” features given their causal relevance. Experimental evaluations on popular classification datasets show that MACFE can improve the pbkp_rediction performance across eight classifiers, outperforms the current state-of-the-art methods on average by at least 6.54%, and obtains an improvement of 2.71% over the best previous works.
Año de publicación:
2022
Keywords:
- Automated machine learning
- Causal feature selection
- Automated feature engineering
Fuente:
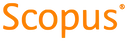
Tipo de documento:
Conference Object
Estado:
Acceso restringido
Áreas de conocimiento:
- Aprendizaje automático
- Algoritmo
Áreas temáticas:
- Ciencias de la computación
- Métodos informáticos especiales