MR images, brain lesions, and deep learning
Abstract:
Featured Application This review provides a critical review of deep/machine learning algorithms used in the identification of ischemic stroke and demyelinating brain diseases. It evaluates their strengths and weaknesses when applied to real world clinical data. Abstract Medical brain image analysis is a necessary step in computer-assisted/computer-aided diagnosis (CAD) systems. Advancements in both hardware and software in the past few years have led to improved segmentation and classification of various diseases. In the present work, we review the published literature on systems and algorithms that allow for classification, identification, and detection of white matter hyperintensities (WMHs) of brain magnetic resonance (MR) images, specifically in cases of ischemic stroke and demyelinating diseases. For the selection criteria, we used bibliometric networks. Of a total of 140 documents, we selected 38 articles that deal with the main objectives of this study. Based on the analysis and discussion of the revised documents, there is constant growth in the research and development of new deep learning models to achieve the highest accuracy and reliability of the segmentation of ischemic and demyelinating lesions. Models with good performance metrics (e.g., Dice similarity coefficient, DSC: 0.99) were found; however, there is little practical application due to the use of small datasets and a lack of reproducibility. Therefore, the main conclusion is that there should be multidisciplinary research groups to overcome the gap between CAD developments and their deployment in the clinical environment.
Año de publicación:
2021
Keywords:
Fuente:
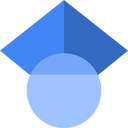
Tipo de documento:
Other
Estado:
Acceso abierto
Áreas de conocimiento:
- Aprendizaje profundo
Áreas temáticas:
- Ciencias de la computación