Machine Learning in Finance: An Application of Pbkp_redictive Models to Determine the Payment Probability of a Client
Abstract:
In the last decades, private debt collection has become an important industry in Ecuador. Debt collection Agencies are organizations that manage the process of collecting money from delinquent debts and their performance is often measured by the collection success rate. However, determining which clients will repay their liabilities is a complex process and many times subjectively judged. Machine learning (ML) models have been successfully implemented on the financing sector for various applications, however a limited amount of work has been published in the pbkp_rediction of a clients debt payment probability. In this study, ML models are trained to pbkp_redict a clients payment probability to an Ecuadorian Debt Collection Agency the first three months after signing a payment agreement. Specifically, a feedforward neural network, logistic regression, and gradient boosting ensemble models are implemented using the SEMMA data mining methodology, which comprises the steps of sample, explore, modify, model, and assess. Furthermore, by analyzing the results of the models, relevant features that determine whether a costumer will pay or not its debt are identified. The results show that neural networks perform better than the competing models in terms of classification accuracy.
Año de publicación:
2022
Keywords:
Fuente:
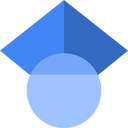
Tipo de documento:
Other
Estado:
Acceso abierto
Áreas de conocimiento:
- Aprendizaje automático
- Finanzas
- Finanzas
Áreas temáticas:
- Economía financiera
- Métodos informáticos especiales
- Dirección general