Machine Learning-Based Indoor Air Quality Baseline Study of the Offices and Laboratories of the Northwest and Southwest Building of Mapúa University - Manila
Abstract:
Indoor Air Quality (IAQ) is one of the utmost concerns when it comes to the health and comfort of the occupants within a structure. However, because of the lack of information with regards to IAQ, especially in the Philippines setting, improvements with respect to the IAQ are not prioritized. Hence, the goal of this study is to determine if the indoor air quality in the Northwest and Southwest buildings of Mapúa University is up to standard set by American Society of Heating, Refrigerating and Air-Conditioning Engineers (ASHRAE) and Occupational Safety and Health (OSH). The study was divided into two phases, one focused on the quantitative walkthrough to evaluate IAQ. This was achieved by using an integrated instrument system using thermohygrometers for temperature and relative humidity, Telaire 7001 for CO2 concentration, and SKC IOM Sampler for particulate matter. Results show that in some rooms, temperature exceeded the range for thermal comfort and majority of the rooms does not comply with the ASHRAE standard for CO2 concentration and the particulate matter remained in the same zone in all of the rooms. The second phase of the study focused on the development of pbkp_redictive models based on the results of the quantitative walkthrough from the first phase. The pbkp_redictive models were used to pbkp_redict the ideal number of occupants in a room given the IAQ parameters. Model performance showed that stochastic gradient boosting (gbm) and support vector machine with Radial Basis Function Kernel (svmRadial) are the best performing models with R2 and RMSE of 0.6838, 0.7777 and 0.812, 0.804 respectively.
Año de publicación:
2020
Keywords:
- support vector machine radial basis function kernel
- quantitative walkthrough
- regression machine learning
- stochastic gradient boosting
- indoor air quality
- baseline model
Fuente:
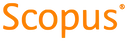
Tipo de documento:
Conference Object
Estado:
Acceso restringido
Áreas de conocimiento:
- Aprendizaje automático
- Contaminación del aire
- Ciencia ambiental
Áreas temáticas:
- Ciencias de la computación
- Tecnología (Ciencias aplicadas)
- Ingeniería sanitaria