Machine Learning-Based Traffic Classification in Software-Defined Networking: A Systematic Literature Review, Challenges, and Future Research Directions
Abstract:
The growing diversification of Internet applications and the continuous evolution of network infrastructure with emerging technologies have complicated network management. Network traffic classification is a key enabler for managing network resources according to the quality of service and security requirements. However, traditional traffic classification methods based on Deep Packet Inspection do not meet the stringent scalability, security, and privacy requirements. The centralized controller of Software-Defined Networking offers a global vision of the network, facilitating traffic analysis and providing direct programming capabilities. Traffic flows can be dynamically adjusted to satisfy the changing network requirements. These characteristics, along with the application of Machine Learning techniques have made it possible to integrate intelligence into networks, optimize them, and better manage and maintain them. In this context, this work aims to provide a Systematic Literature Review on traffic classification in Software-Defined Networking with Machine Learning techniques. Furthermore, we analyze and organize the selected seminal works based on the categorization of traffic classes and the employed Machine Learning techniques to draw meaningful research conclusions. Finally, we identify new challenges and future research directions on this topic.
Año de publicación:
2022
Keywords:
- deep learning
- Softwaredefined networking
- traffic classification
- Machine learning
Fuente:
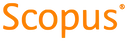
Tipo de documento:
Article
Estado:
Acceso restringido
Áreas de conocimiento:
- Aprendizaje automático
- Ciencias de la computación
Áreas temáticas de Dewey:
- Ciencias de la computación