Machine learning approach to forecasting urban pollution
Abstract:
This work addresses the question of how to pbkp_redict fine particulate matter given a combination of weather conditions. A compilation of several years of meteorological data in the city of Quito, Ecuador, are used to build models using a machine learning approach. The study presents a decision tree algorithm that learns to classify the concentrations of fine aerosols, into two categories (>15μg/m3 vs. <15μg/m3), from a limited number of parameters such as the level of precipitation and the wind speed and direction. Requiring few rules, the resulting models are able to infer the concentration outcome with significant accuracy. This fundamental research intends to be a preliminary step in the development of a web-based platform and smartphone app to alert the inhabitants of Ecuador's capital about the risk to human health, with potential future application in other urban areas.
Año de publicación:
2016
Keywords:
- decision tree
- Pbkp_redictive model
- urban pollution
- fine particulate matter
- Machine learning
Fuente:
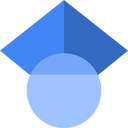
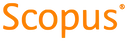
Tipo de documento:
Conference Object
Estado:
Acceso restringido
Áreas de conocimiento:
- Aprendizaje automático
- Contaminación
- Ciencia ambiental
Áreas temáticas de Dewey:
- Métodos informáticos especiales
- Ingeniería sanitaria
- Otros problemas y servicios sociales