Machine learning techniques versus complexity theory in the cerebral haemodynamics of traumatic brain injury patients
Abstract:
The objective of this study was to compare two paradigms of haemodynamic signals analysis which have been used to characterize between two physiological states. Cerebral blood flow velocity and arterial blood pressure signals of 30 patients with traumatic brain injury (TBI) and 30 healthy subjects were obtained non-invasively. Although different machine learning models have been tested with successful results in many cases of clinical interest, there is emerging evidence that complexity and entropy analysis of biomedical signals can detect underlying changes in physiology which relates to diseases. In many studies, both paradigms have been proved in high accuracy in discriminating between health and disease. In this current work a SVM model and two Complexity-Entropy planes were developed, achieving great power in discriminating health from TBI patients, with an AUC of 0.89 for the machine learning approach, and a highest 0.94 AUC by one of the Complexity-Entropy planes. There are almost no cases that compare these two paradigms, which makes it of great interest to put them side by side and discuss their contributions and particularities.
Año de publicación:
2021
Keywords:
- Machine learning
- Traumatic brain injury
- cerebral haemodynamics
- complexity-entropy causality plane
- SUPPORT VECTOR MACHINES
Fuente:
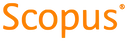
Tipo de documento:
Conference Object
Estado:
Acceso restringido
Áreas de conocimiento:
Áreas temáticas:
- Enfermedades
- Medicina y salud
- Fisiología humana