Machine-Learning-Aided Optimization Framework for Design of Medium-Voltage Grid-Connected Solid-State Transformers
Abstract:
Due to the lack of a comprehensive multiobjective solid-state transformer (SST) design framework, SST designs are mostly obtained through trial/experience. In this article, a machine-learning (ML)-aided optimal SST design framework is proposed, which involves the objectives of maximizing efficiency ( η ) and power density ( ρ ). The challenges of computationally expensive magnetics design, coupled with the correlation between magnetics design and performance of semiconductor devices, are tackled by developing a hybrid local optimization algorithm. This local optimization is subsequently learned through ML techniques, using a limited number of optimal design data sets, and, thus, assists in the genesis of optimal SST design limits for several combinations of semiconductor devices and switching frequencies. The proposed framework is implemented for a cascaded matrix-based dual-active-bridge (CMB-DAB) SST comprising of SiC MOSFETs to demonstrate the optimization routine. The optimization results exhibit low-error fits of the selected ML models and the η - ρ limits in different categories of optimal SST designs. The SiC-based SST designs are also observed to offer better η - ρ optimal designs compared to Si-based SSTs. A laboratory-scale CMB-DAB prototype with experimental measurements is also presented to validate the proposed design optimization framework at a scaled-down level.
Año de publicación:
2021
Keywords:
- solid-state transformer (SST)
- Machine Learning (ML)
- Design Optimization
- Silicon Carbide (SiC)
Fuente:
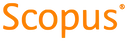
Tipo de documento:
Article
Estado:
Acceso restringido
Áreas de conocimiento:
Áreas temáticas:
- Ciencias de la computación
- Física aplicada
- Métodos informáticos especiales