Machine-learning based approaches for self-tuning trajectory tracking controllers under terrain changes in repetitive tasks
Abstract:
The use of resources in autonomous vehicles when manoeuvring along changing terrain is an issue yet to be faced in the industrial field, such as mining and agriculture, where automated machinery performs repetitive tasks. If the machinery is not capable to overcome such terrain disturbances during its motion, the vehicle spends more energy than the necessary as the motion controller is adapted to the new terramechanical scenario. The latter usually includes a re-tuning of the controller and the corresponding loss of man-hours until obtaining the desired responses. In this context, we propose a self-tuning methodology based on probabilistic approaches and machine learning techniques to improve the performance of the controllers through reducing trajectory tracking errors and control input efforts, as the vehicle repeats its trajectory and learns from the wheel-terrain interaction. Three degree-of-freedom motion controllers are used to test our techniques, although our proposal is not restricted to the nature of such controllers. For the validation of our hypothesis, we considered three tests: one is performed via simulation using a modified kinematic model of the vehicle and slippage constraints, whereas other two extensive trials were carried out in field using an electric vehicle – Twizy, made by Renault – under different types of shaped trajectories and irregular terrain. In particular, two terrains: grass and muddy, and their transitions. The metrics used herein and previously published in the literature have shown that the self-tuning methodologies proposed in this work decreases the trajectory tracking errors up to 18%, saves energy in the effort input of the actuators up 15%, and in general, increases the performance of the controllers up to 22% when compared to efficient manual tuning. The experimental results as well as the statistical analysis of our proposal are presented in detail herein.
Año de publicación:
2018
Keywords:
- Robot self-tuning
- MACHINE-LEARNING
- Energy consumption
- Wheel–terrain interaction
- Motion controller
Fuente:
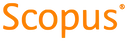
Tipo de documento:
Article
Estado:
Acceso restringido
Áreas de conocimiento:
- Aprendizaje automático
- Sistema de control
Áreas temáticas:
- Métodos informáticos especiales
- Ciencias de la computación
- Física aplicada