A Fuzzy Neural Approach with Multiple Models to Time-Dependent Short Term Power Load Forecasting Based on Weather
Abstract:
With the advancement of the big data technology, making use of the relevant data from different sources to pbkp_redict the power load can become a more feasible task for power system administration. This paper presents a new adaptive neuro-fuzzy inference system (ANFIS) approach with multiple models to pbkp_redict time-dependent power load values based on the weather data collected from different regions. The results show that averaging the pbkp_redicted values of 10 repeated runs generates the best pbkp_rediction results using average weather data as input variables. Thus the load curves with more repeated runs have better general performances. In addition the load curves on holidays show better pbkp_redictability than those on work-days. The results also show that more input records may not improve the pbkp_rediction accuracy. Finally the control surface generated by ANFIS reveals the impact of temperature and vapour pressure on the power load. The paper concludes that the new neuro-fuzzy approach with multiple models is a viable method for power load forecasting based on weather data.
Año de publicación:
2017
Keywords:
Fuente:
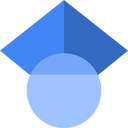
Tipo de documento:
Other
Estado:
Acceso abierto
Áreas de conocimiento:
- Energía
- Aprendizaje automático
- Energía
Áreas temáticas:
- Métodos informáticos especiales
- Física aplicada