Maximum Likelihood identification for Linear Dynamic Systems with finite Gaussian mixture noise distribution
Abstract:
This paper considers the identification of a linear dynamic system driven by a non-Gaussian noise distribution. The noise is approximated by a finite Gaussian mixture, whilst the parameters of the system and the parameters that approximate the noise distribution are simultaneously estimated using the principle of Maximum Likelihood. To this end, a global optimization algorithm is utilized to solve the resulting non-convex optimization problem. It is shown that our approach improves the accuracy of the estimates, when compared with classic estimation techniques such as the pbkp_rediction error method (PEM), in terms of covariance of the estimation error, while also obtaining an approximation of the noise distribution. The benefits of the proposed technique are illustrated by numerical simulations.
Año de publicación:
2019
Keywords:
- Non-Gaussian Noise Distribution
- Maximum likelihood
- Linear Dynamical Systems
- Gaussian Mixture Model
Fuente:
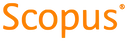
Tipo de documento:
Conference Object
Estado:
Acceso restringido
Áreas de conocimiento:
- Optimización matemática
- Optimización matemática
- Inferencia estadística
Áreas temáticas:
- Física aplicada