A Long Short-Term Memory-Based Interconnected Architecture for Classification of Grasp Types Using Surface-Electromyography Signals
Abstract:
Reliable classification of grasp types from human limbs has become an important aspect used by applications with humanoid robotic systems, because of their high accuracy implementations in human movement replication and detection. Biomedical features extracted from muscular signals are commonly used for this purpose, however, their extraction and usage have been targeted independently, with time series features not even considered in the classification stage. Recently, studies show Deep Neural Networks could obtain the signal's features in their internal architecture and use them directly over a classification task, avoiding all pre-processing steps and improving the obtained accuracy. Therefore, the current study proposes a deep architecture based on LSTM Networks for the classification of 6 grasp types as an end-to-end deep model approach, working with raw surface electromyography signals. Classification accuracy of 99.12% was obtained and compared with previous studies which use different machine learning techniques over the same dataset. Results obtained showed that our model's architecture improves previous results as well as provides a robust solution avoiding overfitting, with an F1-score higher than 99% for all grasp types.
Año de publicación:
2023
Keywords:
- surface muscular signals
- Bidirectional LSTM networks
- Muscles
- grasp types classification
- deep learning
- biomedical signal processing
- Feature Extraction
- classification algorithms
- Electromyography
- time series analysis
- Computer Architecture
Fuente:
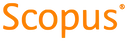
Tipo de documento:
Article
Estado:
Acceso restringido
Áreas de conocimiento:
- Aprendizaje automático
- Ciencias de la computación
Áreas temáticas:
- Métodos informáticos especiales