Mining semantic data for solving first-rater and cold-start problems in recommender systems
Abstract:
Recommender systems are becoming very popular in recent years, mainly in the e-commerce sites, although they are increasing in importance in other areas such as e-learning, tourism, news pages, etc. These systems are endowed with intelligent mechanisms to personalize recommendations about products or services. However, they present some serious drawbacks that impact in user satisfaction. First-rater and cold-start problems are two important drawbacks that take place respectively when new products or new users are introduced in the system. The lack of rating about these products or from these users prevents from making recommendations. Nowadays, traditional collaborative filtering methods have being replaced by web mining techniques in order to deal with scalability and performance problems, but first-rater and cold-start ones require a different strategy. In this work, we propose a methodology that combines data mining techniques with semantic data in order to overcome these two important shortcomings. © 2011 ACM.
Año de publicación:
2011
Keywords:
- Cold-start
- First-rater
- associative classification
- semantic web mining
- recommender systems
Fuente:
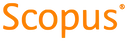
Tipo de documento:
Conference Object
Estado:
Acceso restringido
Áreas de conocimiento:
- Minería de datos
- Ciencias de la computación
Áreas temáticas de Dewey:
- Métodos informáticos especiales