Modeling Wireless Propagation Channel: A Traditional Versus Machine Learning Approach
Abstract:
Wireless networks are one of the most important technologies worldwide, as modeling the different telecommunication channels is an active task of several groups of researchers. Obtaining a theoretical or semi-empirical model of a channel allows improving the planning of wireless networks. The proposed methodology allows channels to be modeled semi-empirically using machine learning tools. This article presents the use of Machine Learning techniques as a tool for describing wireless channels in the 2.4 GHz and 5.8 GHz bands with Zigbee and WiMAX technologies, respectively. This allows to develop a more accurate propagation model, in addition with the generation of heat maps and more reliable schedules. A synthetic data generation technique is proposed, in order to train the Support Vector Machines algorithms of Regression Learning, with this, two semi-empirical models are generated with a lower Root Mean Square Error of 1.4% for Zigbee, and 13,84% for WiMAX, with respect to traditional models.
Año de publicación:
2022
Keywords:
- and Zigbee
- Support Vector Regression
- Wimax
- Heat maps
- synthetic data
- Regression learning
Fuente:
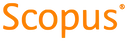
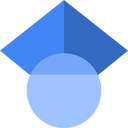
Tipo de documento:
Conference Object
Estado:
Acceso restringido
Áreas de conocimiento:
- Comunicación
- Aprendizaje automático
Áreas temáticas:
- Ciencias de la computación