Modeling diversity in ensembles for time-series pbkp_rediction based on self-organizing maps
Abstract:
A Self Organizing Map (SOM) projects high-dimensional feature vectors onto a low-dimensional space. If an appropriate feature vector is chosen, this ability may be used for measuring and adjusting different levels of diversity in the selection of models for building ensembles. In this paper, we present the results of using a SOM for selecting suitable models in ensembles used for long-term time series pbkp_rediction. The temporal behavior of the pbkp_redictors is represented by feature vectors built with a sequence of the errors achieved in each pbkp_rediction step. Each neuron in the map represents a cluster of models with similar accuracy; the adjustment of diversity between models is achieved by measuring the distance between neurons on the map. Our experiments showed that this strategy generated ensembles with an appropriate level of diversity among their components, obtaining a better performance than just using a unique model.
Año de publicación:
2016
Keywords:
Fuente:
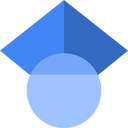
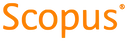
Tipo de documento:
Conference Object
Estado:
Acceso restringido
Áreas de conocimiento:
- Aprendizaje automático
- Simulación por computadora
- Simulación por computadora
Áreas temáticas:
- Ciencias de la computación