Modeling link events in high reliability networks with support vector machines
Abstract:
High reliability communication networks (HRCN) are characterized by very low failure rates, and extremely short unavailability periods. The accurate modeling of the link availability in HRCN is a non-trivial problem, given that an extremely low number of historic events have been observed. We propose a statistical learning model for link event pbkp_rediction in HRCN based on Support Vector Machines (SVM) for nonlinear regression. The model flexibility can be improved by grouping pbkp_redictor variables of different nature. A surrogate data set is made, which mimics the basic properties of links in a real network, and it is used for simulations that yield basic knowledge about the use and performance of the proposed SVM model. A true network example, based on two years of historic data, is also analysed. The proposed SVM model yields better performance than other tested methods (frequentist, and Neural Network estimators), specially during the first years obtaining the historic data in a HRCN, when the number of events is critically low. © 2010 IEEE.
Año de publicación:
2010
Keywords:
- Mercer kernels
- High reliability communication network
- Network link
- Support Vector Machine
- Link events
Fuente:
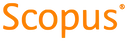
Tipo de documento:
Article
Estado:
Acceso restringido
Áreas de conocimiento:
- Aprendizaje automático
- Ciencias de la computación
- Ciencias de la computación
Áreas temáticas:
- Ciencias de la computación
- Métodos informáticos especiales
- Funcionamiento de bibliotecas y archivos