Modelling of multi-nutrient interactions in growth of the dinoflagellate microalga Protoceratium reticulatum using artificial neural networks
Abstract:
This study examines the use of artificial neural networks as predictive tools for the growth of the dinoflagellate microalga Protoceratium reticulatum. Feed-forward back-propagation neural networks (FBN), using Levenberg-Marquardt back-propagation or Bayesian regularization as training functions, offered the best results in terms of representing the nonlinear interactions among all nutrients in a culture medium containing 26 different components. A FBN configuration of 26-14-1 layers was selected. The FBN model was trained using more than 500 culture experiments on a shake flask scale. Garson's algorithm provided a valuable means of evaluating the relative importance of nutrients in terms of microalgal growth. Microelements and vitamins had a significant importance (approximately 70%) in relation to macronutrients (nearly 25%), despite their concentrations in the culture medium being various orders of magnitude smaller. The approach presented here may be useful for modelling multi-nutrient interactions in photobioreactors. © 2013 Elsevier Ltd.
Año de publicación:
2013
Keywords:
- MICROALGA
- Dinoflagellate
- Artificial Neural Network
- Growth modelling
Fuente:
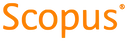
Tipo de documento:
Article
Estado:
Acceso restringido
Áreas de conocimiento:
- Red neuronal artificial
- Simulación por computadora
Áreas temáticas de Dewey:
- Ciencias de la computación
- Microorganismos, hongos y algas
- Física aplicada

Objetivos de Desarrollo Sostenible:
- ODS 2: Hambre cero
- ODS 14: Vida submarina
- ODS 9: Industria, innovación e infraestructura
